Data Annotation in Machine Learning: Boosting Business Efficiency with Keymakr
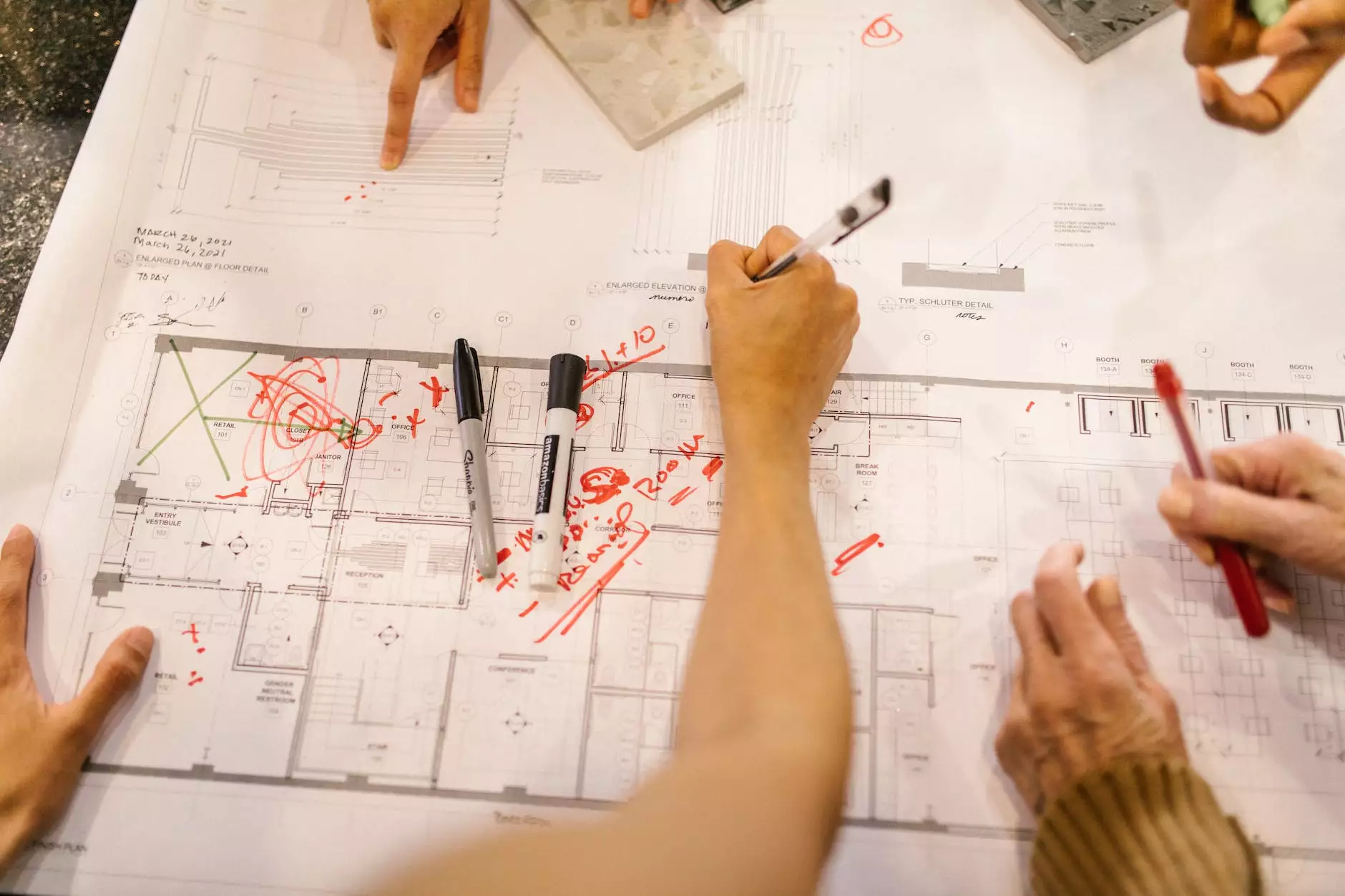
In the modern digital landscape, data annotation has emerged as a vital component of the machine learning ecosystem. As businesses increasingly rely on artificial intelligence (AI) to drive growth and innovation, proper data annotation becomes crucial in enhancing the accuracy and efficiency of AI models. This article dives deep into the realm of data annotation in machine learning, examining its importance for businesses, how it integrates into the services offered by Keymakr.com, and its overarching impact on industries such as Home Services and Locksmiths.
1. Understanding Data Annotation and Its Role in Machine Learning
Data annotation refers to the process of labeling or categorizing data, such as images, text, videos, or audio, to make it comprehensible for machine learning algorithms. By providing a "ground truth" for the machine learning models, data annotation enables these systems to make accurate predictions and classifications. The types of data annotations include:
- Image Annotation: Labeling images with tags, bounding boxes, or segmentation masks.
- Text Annotation: Involves categorizing text into various classes or providing entities with explanations.
- Video Annotation: Tagging specific frames within a video for tasks such as object tracking.
- Audio Annotation: Labeling audio clips for speech recognition or sound classification.
In essence, the data annotation process creates the foundation for accurate AI models that can learn and adapt from various data inputs.
2. Importance of Data Annotation in Business Growth
The significance of data annotation in machine learning cannot be overstated, especially for businesses aiming to leverage AI technologies. Here are key reasons why businesses must invest in high-quality data annotation services:
- Enhanced Accuracy: Properly annotated data leads to more accurate predictions by machine learning models, minimizing mistakes and improving overall performance.
- Scalability: Annotated data can be scaled easily as more data becomes available, facilitating continuous learning for AI systems.
- Improved Decision-Making: With data-driven insights derived from annotated data, businesses can make informed decisions that enhance operations and customer engagement.
- Competitive Advantage: Companies that effectively utilize data annotation can stay ahead of competitors by optimizing their services and offerings through advanced machine learning solutions.
3. Keymakr: Pioneering Data Annotation Services
Keymakr.com specializes in providing top-notch data annotation services tailored to businesses' specific needs. By understanding the critical role that data annotation in machine learning plays, Keymakr offers solutions designed to meet the requirements of various industries, including Home Services and Locksmiths.
Keymakr's data annotation services include:
- Custom Data Annotation: Tailored solutions based on unique business requirements.
- Quality Assurance: Rigorous checks to ensure data quality and usability for machine learning models.
- Rapid Turnaround: Efficient processes that ensure timely delivery of annotated datasets.
- Industry Expertise: Skilled annotators with a deep understanding of various sectors, providing insights that enhance annotation quality.
3.1 Integrating Data Annotation with Home Services
As the Home Services industry continues to evolve, the need for efficient data processing becomes more pronounced. Data annotation can significantly enhance the operational efficiency of companies serving in this domain. For instance, services can leverage annotated images to train AI models on identifying areas that require maintenance or repair.
3.2 Optimizing Locksmith Services with Data Annotation
In the Locksmith industry, data annotation in machine learning can be utilized to manage customer inquiries and optimize service routes. By annotating data related to customer requests and service incidents, locksmiths can leverage machine learning algorithms to predict peak times for service requests, allowing for better resource allocation and enhanced customer service.
4. The Process of Data Annotation
The journey toward obtaining high-quality annotated data is a structured process involving several steps:
- Data Collection: Gather raw data from various sources representing the specific domain.
- Data Preprocessing: Prepare the data for annotation by cleaning and organizing it to eliminate inconsistencies.
- Annotation Guidelines Development: Create clear guidelines that annotators will follow to ensure consistency across annotations.
- Data Annotation: Skilled annotators label the data according to the established guidelines.
- Quality Control: Conduct thorough checks to ensure the accuracy and relevance of annotations.
- Delivery: Provide the annotated dataset in a format compatible with the client's machine learning infrastructure.
5. Challenges in Data Annotation
While the benefits of data annotation are substantial, several challenges can arise during the process:
- Subjectivity: The interpretation of data can vary among annotators, leading to inconsistencies and potential inaccuracies.
- Volume of Data: The increasing amount of data generated necessitates scalable annotation processes to keep up with business demands.
- Costs: High-quality data annotation can be resource-intensive, requiring investment in skilled personnel and tools.
- Time Constraints: Rapidly changing business environments may require faster turnaround times for annotated data.
To address these challenges, partnering with experts like Keymakr.com ensures that businesses can overcome these hurdles effectively and focus on leveraging machine learning to enhance their operations.
6. Future of Data Annotation in Machine Learning
The future of data annotation in machine learning is bright, with ongoing advancements poised to reshape how businesses handle data. Emerging technologies such as automated annotation tools and AI-driven data labeling systems are set to revolutionize the landscape:
- Automated Tools: Algorithms capable of semi-automating the annotation process are being developed, helping to reduce the burden on human annotators.
- Integration with Machine Learning: Automated systems that learn from previous annotations can improve accuracy and efficiency over time.
- Collaboration Between Humans and AI: A hybrid model combining human intelligence with machine capabilities will enhance data annotation processes.
As businesses strive to harness the power of AI, the importance of high-quality data annotation will only continue to grow. Companies that prioritize effective data annotation processes will find themselves well-positioned to lead in their respective industries.
7. Conclusion
In conclusion, the role of data annotation in machine learning is indispensable for businesses looking to leverage AI for growth and innovation. Keymakr.com exemplifies the commitment to quality and efficiency in providing comprehensive data annotation services that cater to diverse industries, including Home Services and Locksmiths. By investing in high-quality annotated data, businesses can unlock the true potential of machine learning, enhancing their decision-making capabilities, improving operational efficiency, and ultimately achieving growth and success in the digital age.
For businesses aiming to improve their machine learning models and take their operations to the next level, partnering with Keymakr for data annotation services is not just a choice; it is a strategic advantage. Start your journey towards enhanced business intelligence today!
data annotation machine learning