The Significance of the **Bounding Box** in Data Annotation
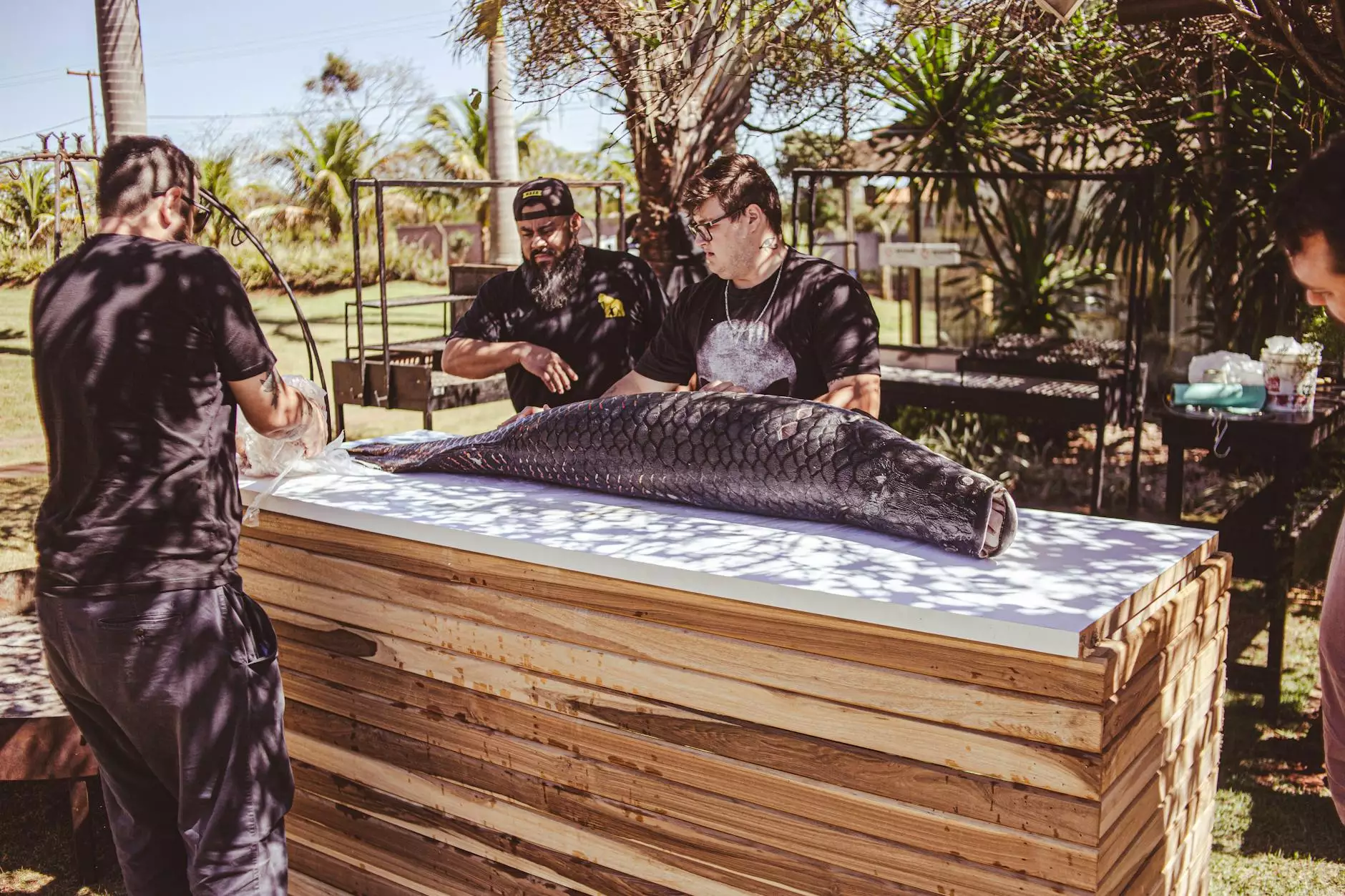
In a rapidly evolving technological landscape, data annotation has become a critical component in training artificial intelligence (AI) and machine learning (ML) models. One of the most prevalent techniques in this domain is the use of the bounding box. This article aims to delve deeply into what a bounding box is, its applications, and the essential role it plays in data annotation tools and platforms, particularly at keylabs.ai.
What is a Bounding Box?
A bounding box is a rectangular box that encapsulates a specified object within an image or video frame. It is defined by two sets of coordinates: the x and y positions of the top-left corner, and the width and height of the rectangle. This simple yet effective visualization method is crucial for object detection tasks in computer vision.
How Bounding Boxes Work
In practical terms, when an image is annotated, the data annotator places a bounding box around the object of interest. This serves multiple purposes:
- Object Detection: By providing the exact location and boundaries of an object, it allows ML models to learn to recognize and classify various entities effectively.
- Training Algorithms: Annotated images with bounding boxes help algorithms learn from labeled data, improving their accuracy in identifying similar objects in new datasets.
- Data Quality: Ensuring precise placement of bounding boxes enhances the overall quality of the dataset, leading to better-performing models.
The Applications of Bounding Boxes in Different Sectors
Bounding boxes have widespread applications across various sectors, including:
1. Autonomous Vehicles
In the realm of autonomous driving, vehicles must identify pedestrians, road signs, and other crucial objects in their environment. Bounding boxes help in accurately locating these objects, enabling vehicles to make informed driving decisions. This accuracy is vital for safety and efficiency on the road.
2. Retail and Inventory Management
In retail settings, bounding boxes are used in stock management and customer behavior analysis. By tracking products on shelves through bounding box annotations, retailers can gather valuable insights into inventory levels and customer interactions, ultimately enhancing the shopping experience.
3. Medical Imaging
In healthcare, bounding boxes are utilized to annotate medical images, such as MRI and CT scans. By marking areas of interest, such as tumors or anomalies, healthcare professionals can train machine-learning models to assist in diagnostics, leading to quicker and more accurate patient assessments.
Why Choose KeyLabs.ai for Your Data Annotation Needs
At KeyLabs.ai, we provide cutting-edge data annotation tools and data annotation platforms designed to streamline the annotation process, especially with the use of bounding boxes. Here’s why we stand out:
1. Robust Annotation Tools
Our tools are intuitive and easy to use, allowing for efficient placement of bounding boxes without sacrificing accuracy. Annotators can adjust bounding boxes seamlessly, ensuring they fit perfectly around objects.
2. Scalability and Flexibility
Whether you have a small project or a large dataset, KeyLabs.ai can accommodate your needs. Our platform scales easily, allowing for massive parallel processing, which is crucial for large-scale data annotation projects.
3. Quality Assurance
We implement stringent quality checks to ensure every bounding box is placed accurately, thus maintaining high data integrity. Our quality assurance teams review annotations to double-check accuracy, providing you with reliable datasets for your AI models.
Best Practices for Creating Accurate Bounding Boxes
To maximize the effectiveness of bounding boxes in your data annotation work, consider the following best practices:
1. Precision is Key
When placing a bounding box, aim for the most precise fit around the object. A box that is too tight or too loose can lead to misclassification.
2. Consistency Matters
Consistency in how bounding boxes are drawn is vital. Establish clear guidelines that annotators should follow to ensure uniformity across the dataset.
3. Use Quality Annotation Tools
Invest in high-quality data annotation software that facilitates easy and accurate placement of bounding boxes. Tools offered by KeyLabs.ai are optimized for this purpose, making the process efficient and effective.
4. Regular Reviews and Feedback
Implement a system for regular reviews of the annotations. Providing feedback to annotators can help improve their accuracy over time.
Conclusion
In conclusion, the bounding box serves as an essential element in the world of data annotation, underpinning many successful applications in AI and machine learning. Its simple structure belies its complexity and impact on the development of intelligent systems. By understanding its functions and best practices, businesses can significantly enhance their annotation efforts, leading to better-trained models and, ultimately, superior products and services.
At KeyLabs.ai, we are dedicated to providing the best data annotation solutions to help your business thrive. Whether you need a streamlined data annotation tool or a robust data annotation platform, look no further than KeyLabs.ai for unparalleled quality and efficiency.